A New Division of Labor: IoT, Wearables and the Human Workforce
The personal computer has had a monumental impact on the productivity of desktop workers. The era of blueprints, typewriters, desk phones, and paper files has given way to CAD, speech recognition, email, and software platforms. Just about every aspect of how work gets done has completely changed in the past three decades, ranging from creation to communication to control.
As in previous generations of technology innovation, the deployment of desktop computers initially required a considerable amount of abstraction and steep learning curves: creating even a simple sketch on a screen required coding and math skills. Experts and many mitigated layers of knowledge were required to effectively use this newly-created work resource. As computers and software evolved, using them became more intuitive, but their users were still tied to desks.
The ensuing era of mobility helped greatly. It unchained the device and led to the creation of wholly new solutions that overcame the challenges of location, real time and visual consumption of the world.
But there is one group that – comparatively speaking – benefited much less from all these changes: the legions of non-desk workers — those on the factory floor, on telephone poles, in mines, on oil rigs or on the farm for whom even a rugged laptop or tablet is impractical or inconvenient. The mobile era unchained desk workers from their desks but its contribution to workers in the field, to the folks who work on things rather than information, was negligible. Working on things often requires both hands to get the job done, and also doesn’t map well to a desktop abstraction.
Enter the wearable device, a new device class enabled by mobile-driven miniaturization of components, the proliferation of affordable sensor technology, and the movement to the cloud.
Wearable devices started as a consumer phenomenon (think smartwatches), mostly built around sensors. Initially, they focused on elevating the utility of the incorporated sensor and their market success was commensurate with how well the sensor data stream could be laddered up to meaningful and personalized insights. With the entrance of the “traditional” mobile actors, wearables’ role expanded into facilitating access, in a simplified way, to the more powerful devices in a user’s possession (e.g., their smartphone). The consumer market for wearables continues to pivot around the twin notions of access and self-monitoring. However, to understand the deeper and longer-term implications of the emergence of intelligent wearable devices, we need to look to the industrial world.
An important, new chapter in wearable history was written by Google Glass, the first affordable commercial Head-Mounted Display (HMD). Although it failed as a consumer device, it successfully catalyzed the introduction of HMDs in the enterprise. Perhaps even more importantly, this new device type led the way in integrating with other enterprise systems, aggregating the compute power of a node and the cloud – centered on a wearer. Unlike the shift to mobile devices, however, this has the potential to drive profound changes in the lives of field workers and could be a harbinger of even deeper changes in how all of us interact with the digital world.
Division of Labor: Re-empowering the Human Workforce
Computers and handheld devices had a limited impact on non-desk workers. But technological changes such as automation, robotics, and the Internet of Things (IoT) had a profound impact, effectively splitting the industrial world into work that is fit for robots and work that isn’t. And the line demarcating this division itself is in continuous motion.
Early robotic systems focused on automating precise, repetitive, and often physically demanding activities. More recent advances in analytics and decision support technology (e.g., Machine Learning and Artificial Intelligence [AI]) and integration via IoT have led to the extension of physical robots into the digital domain, coupling them with software counterparts (software agents, bots, etc.) capable of more dynamic response to the world around them. Automation is thus becoming more autonomous and, as it does so, it’s increasingly moving out of its isolated, tightly controlled confines and becoming ever more entwined with human activity.
Because automation inherently displaces human participation in industrial processes, the rapid advances in analytics, complex event processing, and digital decision-making have prompted concerns about the possibility of “human obsolescence.” In terms of the role of bulk labor, this is a real concern. However, the AI community has perpetually underestimated the sophistication of the human brain and the limits to AI-based machine autonomy in the real world have remained clear: creativity, decision-making, complex, non-repetitive activity, untrainable pattern recognition, self-directed evolution, and intuition are still largely the domains of the human workforce, and are likely to remain so for some time.
Even the most sophisticated autonomous machines can only operate in a highly constrained environment. Self-driving vehicles, for example, depend on well-marked, regular roads and the goal of an “unattended autonomous vehicle” is very likely to require extensive orchestration and physical infrastructure, and the resolution of some very serious security challenges. By contrast, the human brain is extraordinarily well adapted to operating in the extreme fuzziness of the real world and is a marvel of efficiency. Rather than try to replace it with fully digital processes, a safer, and more cost-effective strategy would be to find ever better and closer ways to integrate human processing with the digital world. The role of wearable technology provides a first path forward in this regard.
Initial industrial use cases for wearables have tended to emphasize human productivity through the incorporation of monitoring and “field appropriate” access to task-specific information. The first use cases included training and enabling less experienced field personnel to operate with less guidance and oversight. Some good examples are Librestream’s Onsight which creates “virtual experts,” Ubimax’s X-pick that guides warehouse pickers, or Atheer’s AR-Training solutions. Honeywell’s Connected Plant solution goes a step beyond: it is an “Industrial Internet of Things (IIoT) style” platform that already connects industrial assets and processes for diagnostic and maintenance purposes, a new dimension of value.
The introduction of increasingly robust autonomous machines and the consideration of productivity and monitoring across more complex use cases involving multiple workers and longer spans of time will drive the next generation of use cases.
Next Reality
Consider the following – still hypothetical, although reality based – use case:
Iron ore mining is a complex operation involving machines (some of which are very large), stationary objects and human workers – all sharing the same confined space with limited visibility. It is critical not only to be able to direct the flow of these participants for safety reasons but also to optimize it for maximum productivity.
The first step in accomplishing this requires deploying sensors at the edge that create awareness of context: state, condition, location. Sensors on large machines or objects are not new and increasingly, miners carry an array of sensors built into their hard hats, vests, and wrist-worn devices. But “sense” is not enough – optimization requires a change in behavior. For this, a feedback loop is needed, which is comparatively easy to accomplish with machines. For workers, a display mounted on the hard hat, and haptic actuators embedded in their vest and wrist devices close the feedback loop.
Thus equipped, both human and machine participants in the mining ecosystem can be continuously aware of each other, getting a heads up – or even a warning – about proximity. Beyond awareness, this also allows for independent action: for example, stopping vehicles or giving directional instructions via the HMD or haptic feedback.
Being connected in this way helps to promote safety, but isn’t enough for optimization. For that a backend system that uses historical data, rules and ML algorithms to predict and ultimately prescribe optimum paths is required. This provides humans with key decision support capabilities and a means to provide guidance to machines without explicitly having to operate them. Practically speaking: they operate machines via their presence. Considering the confined environment, this means that sometimes the worker needs to give way to the 50-ton hauler and other times the other way around. What needs to happen gets deduced from the actual conditions, decided in real time, on the edge.
As this use case illustrates, wearable devices are emerging as a new way for humans to interact with machines (physical or digital). The sensors on these devices are also being used in a new and more dynamic way. Whereas each sensor in a traditional industrial context provides a very tightly defined window into a specific operating parameter of a specific asset, sensor data in the emerging paradigm is interpreted situationally. Temperature, speed, vibration may carry very different meanings depending on the task and situation at hand. The Key Performance Indicators (KPIs) to be extracted from these data streams are also task- and situation-specific, as are the ways in which these KPIs are used to validate, certify, and optimize both the individual tasks and the overarching process or mission in which these tasks are embedded.
A key takeaway in considering this new human-machine interaction paradigm is that almost everything is dynamic and situational. And, at least in the industrial context, the logical container for managing all of this is what we’re calling the “Mission.” This has important ramifications for considering what systems need to be in place to enable workers and machines to interoperate in this way and to make possible an IIoT that effectively leverages the unique features of the human brain.
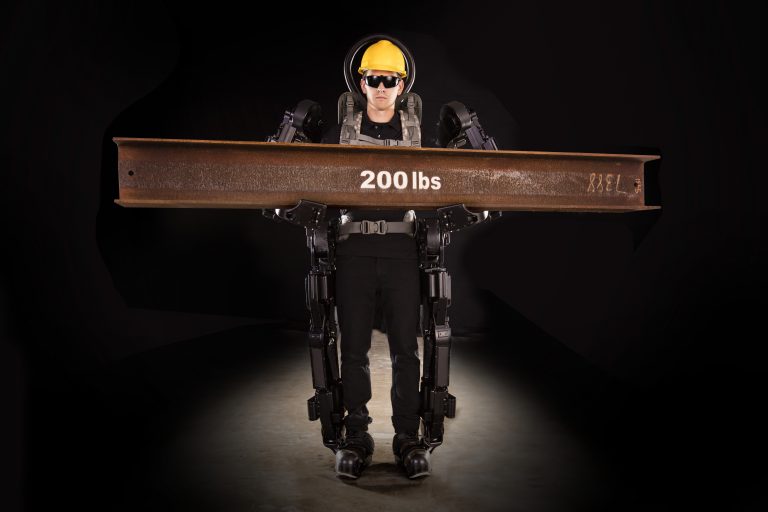
A bit about the authors:
Keith Deutsch is an experienced CTO, VP Engineering, Chief Architect based in the San Francisco Bay area. Peter Orban, based out of New York, leads, builds and supports experience-led, tech-driven organizations globally to grow the share of problems they can solve effectively.